Introduction: Why Parallel Scripting?
Swift is a simple scripting language for executing many instances of ordinary application programs on distributed parallel resources. Swift scripts run many copies of ordinary programs concurrently, using statements like this:
foreach protein in proteinList { runBLAST(protein); }
Swift acts like a structured "shell" language. It runs programs concurrently as soon as their inputs are available, reducing the need for complex parallel programming. Swift expresses your workflow in a portable fashion: The same script runs on grids like OSG, as well as on multicore computers, clusters, clouds, and supercomputers.
In this tutorial, you’ll be able to first try a few Swift examples (parts 1-3) on the OSG Connect login host, to get a sense of the language. Then in parts 4-6 you’ll run similar workflows on distributed OSG Connect resources, and see how more complex workflows can be expressed with Swift scripts.
Workflow tutorial setup
To get started, do:
$ cd $HOME $ tutorial swift $ cd osg-swift
Verify your environment
To verify that Swift (and the Java environment it requires) are working, do:
$ java -version # verify that you have Oracle JAVA 1.6 or later $ swift -version # verify that you have Swift 0.94.1 (RC2 revision)
![]() |
If you re-login or open new ssh sessions, you will need to re-run source setup.sh in each ssh window: |
$ cd $HOME/osg-swift # change to the newly created tutorial directory $ source setup.sh # sets PATH and swift config files
To check out the tutorial scripts from SVN
If you later want to get the most recent version of this tutorial from the Swift Subversion repository, do:
$ svn co https://svn.ci.uchicago.edu/svn/vdl2/SwiftTutorials/OSG-Swift
This will create a directory called "OSG-Swift" which contains all of the files used in this tutorial.
Simple "science applications" for the workflow tutorial
This tutorial is based on two simple example programs (both implemented as bash shell scripts) that serve a very simple stand-ins for real science applications: simulation.sh and stats.sh.
simulation.sh
The simulation.sh script serves as a trivial substitute for a complex scientific simulation application. It generates and prints a set of one or more random integers in the range [0-2^62) as controlled by its command line arguments, which are:
$ ./app/simulate.sh --help ./app/simulate.sh: usage: -b|--bias offset bias: add this integer to all results [0] -B|--biasfile file of integer biases to add to results [none] -l|--log generate a log in stderr if not null [y] -n|--nvalues print this many values per simulation [1] -r|--range range (limit) of generated results [100] -s|--seed use this integer [0..32767] as a seed [none] -S|--seedfile use this file (containing integer seeds [0..32767]) one per line [none] -t|--timesteps number of simulated "timesteps" in seconds (determines runtime) [1] -x|--scale scale the results by this integer [1] -h|-?|?|--help print this help $
All of thess arguments are optional, with default values indicated above as [n].
With no arguments, simulate.sh prints 1 number in the range of 1-100. Otherwise it generates n numbers of the form (R*scale)+bias where R is a random integer. By default it logs information about its execution environment to stderr. Here’s some examples of its usage:
$ simulate.sh 2>log 5 $ head -4 log Called as: /home/wilde/swift/tut/CIC_2013-08-09/app/simulate.sh: Start time: Thu Aug 22 12:40:24 CDT 2013 Running on node: login01.osgconnect.net $ simulate.sh -n 4 -r 1000000 2>log 239454 386702 13849 873526 $ simulate.sh -n 3 -r 1000000 -x 100 2>log 6643700 62182300 5230600 $ simulate.sh -n 2 -r 1000 -x 1000 2>log 565000 636000 $ time simulate.sh -n 2 -r 1000 -x 1000 -t 3 2>log 336000 320000 real 0m3.012s user 0m0.005s sys 0m0.006s
stats.sh
The stats.sh script serves as a trivial model of an "analysis" program. It reads N files each containing M integers and simply prints the average of all those numbers to stdout. Similarly to simulate.sh it logs environmental information to the stderr.
$ ls f* f1 f2 f3 f4 $ cat f* 25 60 40 75 $ stats.sh f* 2>log 50
Basic of the Swift language with local execution
A Summary of Swift in a nutshell
-
Swift scripts are text files ending in .swift The swift command runs on any host, and executes these scripts. swift is a Java application, which you can install almost anywhere. On Linux, just unpack the distribution tar file and add its bin/ directory to your PATH.
-
Swift scripts run ordinary applications, just like shell scripts do. Swift makes it easy to run these applications on parallel and remote computers (from laptops to supercomputers). If you can ssh to the system, Swift can likely run applications there.
-
The details of where to run applications and how to get files back and forth are described in configuration files separate from your program. Swift speaks ssh, PBS, Condor, SLURM, LSF, SGE, Cobalt, and Globus to run applications, and scp, http, ftp, and GridFTP to move data.
-
The Swift language has 5 main data types: boolean, int, string, float, and file. Collections of these are dynamic, sparse arrays of arbitrary dimension and structures of scalars and/or arrays defined by the type declaration.
-
Swift file variables are "mapped" to external files. Swift sends files to and from remote systems for you automatically.
-
Swift variables are "single assignment": once you set them you can’t change them (in a given block of code). This makes Swift a natural, "parallel data flow" language. This programming model keeps your workflow scripts simple and easy to write and understand.
-
Swift lets you define functions to "wrap" application programs, and to cleanly structure more complex scripts. Swift app functions take files and parameters as inputs and return files as outputs.
-
A compact set of built-in functions for string and file manipulation, type conversions, high level IO, etc. is provided. Swift’s equivalent of printf() is tracef(), with limited and slightly different format codes.
-
Swift’s foreach {} statement is the main parallel workhorse of the language, and executes all iterations of the loop concurrently. The actual number of parallel tasks executed is based on available resources and settable "throttles".
-
In fact, Swift conceptually executes all the statements, expressions and function calls in your program in parallel, based on data flow. These are similarly throttled based on available resources and settings.
-
Swift also has if and switch statements for conditional execution. These are seldom needed in simple workflows but they enable very dynamic workflow patterns to be specified.
We’ll see many of these points in action in the examples below. Lets get started!
Part 1: Run a single application under Swift
The first swift script, p1.swift, runs simulate.sh to generate a single random number. It writes the number to a file.
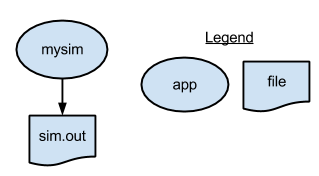
1 type file; 2 3 app (file o) mysim () 4 { 5 simulate stdout=@filename(o); 6 } 7 8 file f <"sim.out">; 9 f = mysim();
The sites.xml file included in each part directory gives Swift information about the machines we will be running on. It defines things like the work directory, the scheduler to use, and how to control parallelism. The sites.xml file below will tell Swift to run on the local machine only, and run just 1 task at a time.
1 <?xml version="1.0" encoding="UTF-8"?> 2 <config xmlns="http://www.ci.uchicago.edu/swift/SwiftSites"> 3 <pool handle="localhost"> 4 <execution provider="local" /> 5 <profile namespace="karajan" key="jobThrottle">0</profile> 6 <profile namespace="karajan" key="initialScore">10000</profile> 7 <filesystem provider="local"/> 8 <workdirectory>.swift/tmp</workdirectory> 9 <profile namespace="swift" key="stagingMethod">local</profile> 10 </pool> 11 </config>
The app file translates from a Swift app function to the path of an executable on the file system. In this case, it translates from "simulate" to simulate.sh and assumes that simulate.sh will be available in your $PATH.
1 localhost simulate simulate.sh 2 persistent-coasters simulate simulate.sh
To run this script, run the following command:
$ cd part01 $ swift p1.swift
The simulate application gets translated to simulate.sh within the apps file.
![]() |
Since the file you created is not named, swift will generate a random name for the file in a directory called _concurrent. To view the created output, run "cat _concurrent/*" |
To cleanup the directory and remove all outputs, run:
$ ./clean.sh
Part 2: Running an ensemble of many apps in parallel with "foreach" loops
The p2.swift script introduces the foreach loop. This script runs many simulations. The script also shows an example of naming the files. The output files are now called sim_N.out.
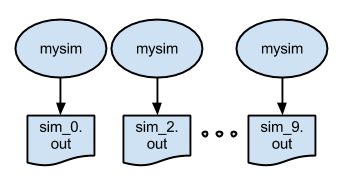
In part 2, we also update the apps file. Instead of using shell script (simulate.sh), we use the equivalent python version (simulate.py). The new apps file now looks like this:
localhost simulate simulate.py
Swift does not need to know anything about the language an application is written in. The application can be written in Perl, Python, Java, Fortran, or any other language.
1 type file; 2 3 app (file o) mysim () 4 { 5 simulate stdout=@filename(o); 6 } 7 8 foreach i in [0:9] { 9 file f <single_file_mapper; file=@strcat("output/sim_",i,".out")>; 10 f = mysim(); 11 } 12
To run the script:
$ cd ../part02 $ swift p2.swift
Part 3: Analyzing results of a parallel ensemble
p3.swift introduces a postprocessing step. After all the parallel simulations have completed, the files created by simulation.sh will be averaged by stats.sh.
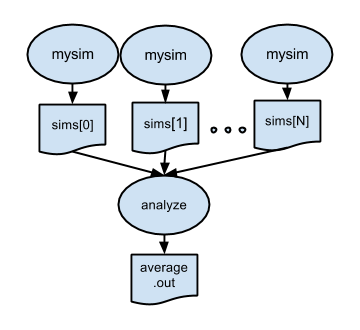
1 2 type file; 3 4 app (file o) mysim (int sim_steps, int sim_values) 5 { 6 simulate "--timesteps" sim_steps "--nvalues" sim_values stdout=@filename(o); 7 } 8 9 app (file o) analyze (file s[]) 10 { 11 stats @filenames(s) stdout=@filename(o); 12 } 13 14 int nsim = @toInt(@arg("nsim","10")); 15 int steps = @toInt(@arg("steps","1")); 16 int values = @toInt(@arg("values","5")); 17 18 file sims[]; 19 20 foreach i in [0:nsim-1] { 21 file simout <single_file_mapper; file=@strcat("output/sim_",i,".out")>; 22 simout = mysim(steps,values); 23 sims[i] = simout; 24 } 25 26 file stats<"output/average.out">; 27 stats = analyze(sims); 28
To run:
$ cd part03 $ swift p3.swift
Running applications on OSG Connect resources with Swift
Part 4: Running a parallel ensemble on OSG Connect resources
p4.swift is the first script that will submit jobs to remote site nodes for analysis. It is similar to earlier scripts, with a few minor exceptions. To generalize the script for other types of remote execution (e.g., when no shared filesystem is available to the compute nodes), the application simulate.sh will get transferred to the worker node by Swift, in the same manner as any other input data file.
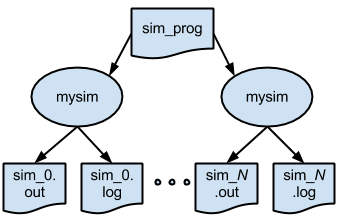
1 2 type file; 3 4 # Application program to be called by this script: 5 6 file simulation_prog <"app/simulate.sh">; 7 8 # "app" function for the simulation application: 9 10 app (file out, file log) simulation (file prog, int timesteps, int sim_range) 11 { 12 sh @prog "-t" timesteps "-r" sim_range stdout=@out stderr=@log; 13 } 14 15 # Command line parameters to this script: 16 17 int nsim = @toInt(@arg("nsim", "10")); # number of simulation programs to run 18 int range = @toInt(@arg("range", "100")); # range of the generated random numbers 19 20 # Main script and data 21 22 int steps=3; 23 24 tracef("\n*** Script parameters: nsim=%i steps=%i range=%i \n\n", nsim, steps, range); 25 26 foreach i in [0:nsim-1] { 27 file simout <single_file_mapper; file=@strcat("output/sim_",i,".out")>; 28 file simlog <single_file_mapper; file=@strcat("output/sim_",i,".log")>; 29 (simout,simlog) = simulation(simulation_prog, steps, range); 30 } 31
To run:
$ swift p4.swift
Output files will be named output/sim_N.out.
In order to run on OSG compute nodes, sites.xml was modified. Here is the new sites.xml we are using for this example. Note the changes between the sites.xml file in this example which specifies "execution provider=condor", and the sites.xml file in part 1, which runs locally by specifying "execution provider=local".
1 2 <config> 3 <pool handle="osg"> 4 <execution provider="coaster" jobmanager="local:condor"/> 5 <profile namespace="karajan" key="jobThrottle">5.00</profile> 6 <profile namespace="karajan" key="initialScore">10000</profile> 7 <profile namespace="globus" key="jobsPerNode">1</profile> 8 <profile namespace="globus" key="maxtime">3600</profile> 9 <profile namespace="globus" key="maxWalltime">00:01:00</profile> 10 <profile namespace="globus" key="highOverAllocation">10000</profile> 11 <profile namespace="globus" key="lowOverAllocation">10000</profile> 12 <profile namespace="globus" key="internalHostname"></profile> 13 <profile namespace="globus" key="slots">20</profile> 14 <profile namespace="globus" key="maxNodes">1</profile> 15 <profile namespace="globus" key="nodeGranularity">1</profile> 16 <workdirectory>.</workdirectory> <!-- Alt: /tmp/swift/OSG/{env.USER} --> 17 <!-- For UC3: --> 18 <profile namespace="globus" key="condor.+AccountingGroup">"group_friends.{env.USER}"</profile> 19 <!-- For OSGConnect --> 20 <profile namespace="globus" key="condor.+ProjectName">"Swift"</profile> 21 <profile namespace="globus" key="jobType">nonshared</profile> 22 23 </pool> 24 </config>
Below is the updated apps file. Since Swift is staging shell scripts remotely to nodes on the cluster, the only application you need to define here is the shell.
1 osg sh /bin/bash
Part 5: Controlling where applications run
p5.swift introduces a postprocessing step. After all the parallel simulations have completed, the files created by simulation.sh will be averaged by stats.sh. This is similar to p3, but all app invocations are done on remote nodes with Swift managing file transfers.
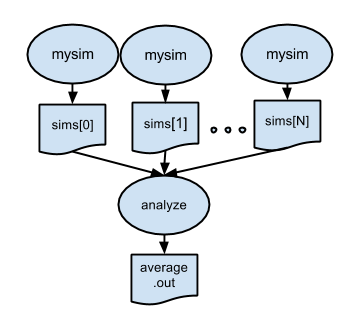
1 type file; 2 3 # Define external application programs to be invoked 4 5 file simulation_prog <"app/simulate.sh">; 6 file analysis_prog <"app/stats.sh">; 7 8 app (file out, file log) simulation 9 (file prog, int timesteps, int sim_range, 10 file bias_file, int scale, int sim_count) 11 { 12 sh @prog "-t" timesteps "-r" sim_range "-B" @bias_file 13 "-x" scale "-n" sim_count 14 stdout=@out stderr=@log; 15 } 16 17 app (file out) analyze (file prog, file s[]) 18 { 19 sh @filename(prog) @filenames(s) stdout=@filename(out); 20 } 21 22 # Command line params to this script 23 24 int nsim = @toInt(@arg("nsim", "10")); # number of simulation programs to run 25 int steps = @toInt(@arg("steps", "1")); # number of "steps" each simulation (==seconds of runtime) 26 int range = @toInt(@arg("range", "100")); # range of the generated random numbers 27 int count = @toInt(@arg("count", "10")); # number of random numbers generated per simulation 28 29 # Perform nsim "simulations" 30 31 tracef("\n*** Script parameters: nsim=%i steps=%i range=%i count=%i\n\n", nsim, steps, range, count); 32 33 file sims[]; # Array of files to hold each simulation output 34 file bias<"bias.dat">; # Input data file to "bias" the numbers: 35 # 1 line: scale offset ( N = n*scale + offset) 36 foreach i in [0:nsim-1] { 37 file simout <single_file_mapper; file=@strcat("output/sim_",i,".out")>; 38 file simlog <single_file_mapper; file=@strcat("output/sim_",i,".log")>; 39 (simout, simlog) = simulation(simulation_prog, steps, range, bias, 100000, count); 40 sims[i] = simout; 41 } 42 43 # Generate "analysis" file containing average of all "simulations" 44 45 file stats<"output/stats.out">; 46 stats = analyze(analysis_prog,sims);
To run:
$ swift p5.swift
Part 6: Specifying more complex workflow patterns
p6.swift build on p5.swift, but adds new apps for generating a random seed and a random bias value.
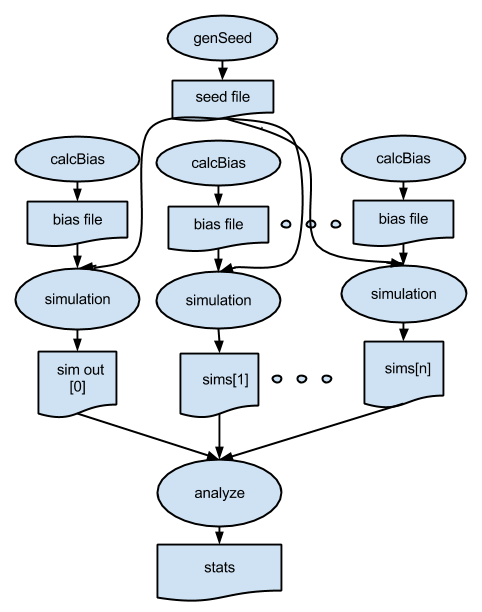
1 type file; 2 3 # Define external application programs to be invoked 4 5 file simulation_prog <"app/simulate.sh">; 6 file analysis_prog <"app/stats.sh">; 7 file genbias_prog = simulation_prog; 8 file genseed_prog = simulation_prog; 9 10 # app() functions for application programs to be called: 11 12 app (file out) genseed (file prog, int nseeds) 13 { 14 sh @prog "-r" 2000000 "-n" nseeds stdout=@out; 15 } 16 17 app (file out) genbias (file prog, int bias_range, int nvalues) 18 { 19 sh @prog "-r" bias_range "-n" nvalues stdout=@out; 20 } 21 22 app (file out, file log) simulation (file prog, int timesteps, int sim_range, 23 file bias_file, int scale, int sim_count, 24 file seed_file) 25 { 26 sh @prog "-t" timesteps "-r" sim_range "-B" @bias_file "-x" scale 27 "-n" sim_count "-S" @seed_file stdout=@out stderr=@log; 28 } 29 30 app (file out) analyze (file prog, file s[]) 31 { 32 sh @prog @filenames(s) stdout=@out; 33 } 34 35 # Command line arguments 36 37 int nsim = @toInt(@arg("nsim", "10")); # number of simulation programs to run 38 int range = @toInt(@arg("range", "100")); # range of the generated random numbers 39 int count = @toInt(@arg("count", "10")); # number of values generated per simulation 40 int steps = @toInt(@arg("steps", "1")); # number of timesteps (seconds) per simulation 41 42 # Main script and data 43 44 tracef("\n*** Script parameters: nsim=%i range=%i count=%i\n\n", nsim, range, count); 45 46 file seedfile<"output/seed.dat">; # Dynamically generated bias for simulation ensemble 47 seedfile = genseed(genseed_prog, 1); 48 49 file sims[]; # Array of files to hold each simulation output 50 51 foreach i in [0:nsim-1] { 52 file biasfile <single_file_mapper; file=@strcat("output/bias_",i,".dat")>; 53 file simout <single_file_mapper; file=@strcat("output/sim_",i,".out")>; 54 file simlog <single_file_mapper; file=@strcat("output/sim_",i,".log")>; 55 biasfile = genbias(genbias_prog, 1000, 20); 56 (simout,simlog) = simulation(simulation_prog, steps, range, biasfile, 100000, count, seedfile); 57 sims[i] = simout; 58 } 59 60 file stats<"output/stats.out">; # Final output file: average of all "simulations" 61 stats = analyze(analysis_prog,sims);
Use the command below to specify the time for each simulation.
$ cd ../part06 $ swift p6.swift -steps=3 # each simulation takes 3 seconds